TAU researchers use AI to understand how cells respond to drug treatments
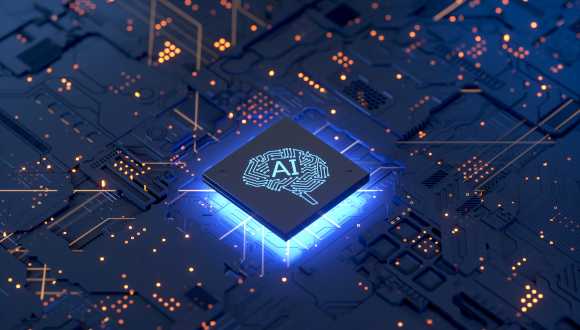
New method enables identification of important biological patterns
Support this researchResearchers from Tel Aviv University (TAU) have developed an innovative method that can help to understand better how cells behave in changing biological environments, such as those found within a cancerous tumor.
The new system, called scNET, combines information on gene expression at the single-cell level with information on gene interactions, enabling the identification of important biological patterns such as responses to drug treatments. It may improve medical research and assist in the development of treatments for diseases.
The research was led by PhD student Ron Sheinin under the supervision of Professor Asaf Madi of TAU’s Faculty of Medical & Health Sciences and Professor Roded Sharan, head of TAU’s School of Computer Science and AI. It was published in Nature Methods on March 17, 2025.
Advanced sequencing technologies allow the measurement of gene expression at the single-cell level. For the first time, researchers can investigate the gene expression profiles of different cell populations within a biological sample and discover their effects on the functional behavior of each cell type. One example is understanding the impact of cancer treatments – not only on the cancer cells themselves but also on the pro-cancer supporting cells or, alternatively, anti-cancer cell populations, such as some cells of the immune system surrounding the tumor.
Despite the high resolution, these measurements are characterized by high levels of noise, which makes it difficult to identify precise changes in genetic programs that underlie vital cellular functions. This is where scNET comes into play.
“scNET integrates single-cell sequencing data with networks that describe possible gene interactions, much like a social network, providing a map of how different genes might influence and interact with each other,” Sheinin says. “scNET enables more accurate identification of existing cell populations in the sample. Thus, it is possible to investigate the common behavior of genes under different conditions and to expose the complex mechanisms that characterize the healthy state or response to treatments.”
“In this research, we focused on a population of T cells, immune cells known for their power to fight cancerous tumors,” Professor Madi adds. “scNET revealed the effects of treatments on these T cells and how they became more active in their cytotoxic activity against the tumor, something that was not possible to discover before due to the high level of noise in the original data.”
“This is an excellent example of how artificial intelligence tools can help decipher biological and medical data, allowing us to gain new and significant insights,” Professor Sharan says. “The idea is to provide biomedical researchers with computational tools that will aid in understanding how the body’s cells function, thereby identifying new ways to improve our health.”
In conclusion, scNET demonstrates how the combination of AI with biomedical research could lead to the development of new therapeutic approaches, reveal hidden mechanisms in diseases, and propose new treatment options.